Over the last four years, I’ve had conversations about data science, machine learning, ethics and the law on several continents. This has included startups, big companies, governments, academics, and nonprofits. And over that time, some patterns are starting to emerge.
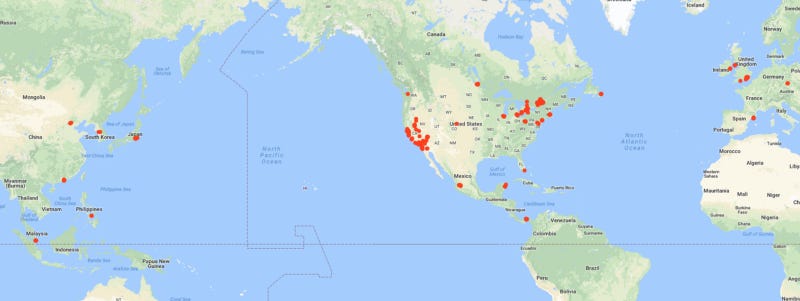
This was last year, and I didn’t have location services turned on all the time.
I’m going to be making some sweeping generalizations in this post. Everyone is different; every circumstance is somehow unique. But in digging into these patterns with colleagues, friends, and audiences both at home and abroad, they reflect many of the concerns of those cultures.
Briefly: In China they worry about the veracity of the data. In Europe they worry about the storage and analysis. And in North America they worry about unintended consequences of acting on it.
Let me dig into those a bit more, and explain how I think external factors influence each.
Data veracity
If you don’t trust your data, everything you build atop it is a house of cards. When I’ve spoken about Lean Analytics or Data Science and Critical Thinking in China, many of the questions are about knowing whether the data is real or genuine.
China is a country in transition. A recent talk by Xi Jinping outlined a plan in which the country creates things first, rather than copying. They want to produce the best students, rather than send them abroad. They’re transitioning from a culture of mimicry and cheap copies to one of leadership and innovation. Just look at their policies on electric cars, or their planned cities, or the dominance of Wechat as a ubiquitous payment system.
When I was in Paris a few years ago, I visited Les Galleries Lafayette, an over-the-top mall whose gold decor and outlandish ornamentation is a paeon to all things commercial. Outside one of the high-end retail outlets was a long queue of Chinese tourists, being let in to buy a purse a few at a time.
As each person completed their purchase, they’d pause at the exit and take a picture of themselves with their new-found luxury item, in front of the store logo. I asked the busdriver what was going on. “They want proof it’s the real,” he replied.
Proof it’s the real.
In a country with a history of copying, where data is conflated with propaganda and competition is relatively unregulated, it’s no wonder veracity is in question.
There are many things a data analyst can do to test whether data is real. One of the most interesting is Benford’s Law, which states that natural data of many kinds follows a power curve. In a random sample of that data, there will be more numbers beginning with a one than a two, more with a two than a three, and so on. It seems like a magic trick, but it’s been used to expose fraud in many fascinating cases.
There are also promising technologies that distribute trust, and tamper-evident sensors, and so on.
But in an era of fake news and truthiness—which is only going to get worse as we start to create fiction indistinguishable from the truth—knowing you’re starting with what’s real is the first step in modern critical thinking.
Storage and Analysis
At a cloud computing event in DC several years ago, I sat at dinner with a French diplomat. Part of the EU parliament, he was in charge of data privacy. “Do you know why the French hate traffic cameras?” he asked me. “Because we can overlook a smudge of lipstick or a whiff of cologne on our partners’ shirts. But we can’t ignore a photograph of them in a car with a lover.”
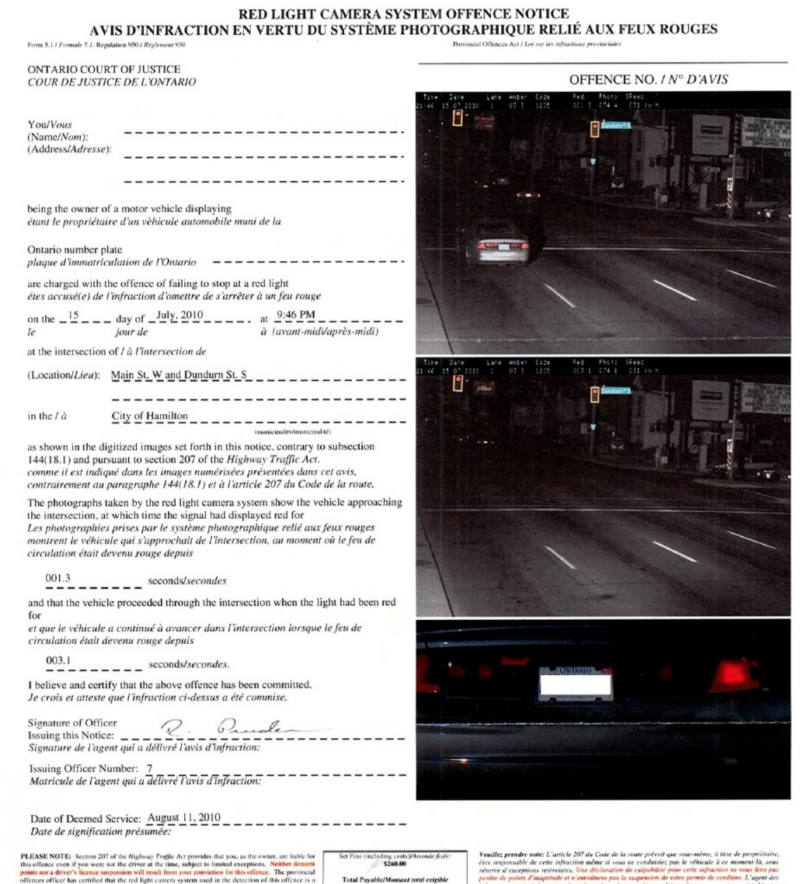
An infraction from Ontario. I couldn’t find a French one. Damned privacy laws.
Indeed, the French amended the laws regarding traffic camera evidence, only sending a photo when a dispute occurs. As he pointed out, “French society functions in the gray areas of legality. Data is too black and white.”
Another European speaker at a separate event talked about data privacy laws, and how information must be protected from the government itself, even when the government stores it. A member of the audience challenged him on this, to which he replied, “you’re from America. You haven’t had tanks roll in, take all the records on citizens, find the Jews, and round them up.” Close borders and the echoes of war inform data storage policy in Europe.
The arrival of GDPR in Europe — with wide-ranging effects beyond, given the global nature of most large companies — is in part an attempt by Europe to exert some control over the technical nation-states. GAFAM (Google, Amazon, Facebook, Apple, and Microsoft) are all US companies; the only close competitors are Baidu, Alibaba, and Tencent, all Chinese. If populations made nations, these would be some of the biggest countries on earth, and Europe doesn’t even have an embassy. GDPR forces these firms to answer the door when Europe comes knocking.
But at the same time, GDPR is a reflection of European concerns, informed by history and culture, of how data should be used, and the fact that we should be its stewards, not the other way around. Nobody should know more about us than we do.
Unintended consequences
The Sloan Foundation’s Daniel Goroff worked on energy nudge policy for the federal government, trying to convince people to consume less electricity, particularly during the warmer months when air conditioning use skyrockets.
Social scientists know that you can use peer pressure to encourage behaviours. For example, if you ask someone to re-use the towel in their hotel room, there’s a certain likelihood that they will. But if you tell them that other guests re-use their towels, they’re about 25% more likely to do so.
Applying this kind of policy to energy conservation makes sense, so utilities send letters to their customers showing them how they’re doing on energy conservation compared to their neighbours, congratulating the frugal and showing the wasteful they can do better.
The problem is, this doesn’t always work. It turns out that if you tell a democrat/liberal they’re consuming more than others, they’ll reduce their consumption as you’d hoped. But if you tell a republican/conservative they’re consuming less, they will increase their consumption so they get their fair share.
Political insight aside, this is a critical lesson: Knowing what the data tells you isn’t the same as using it to produce the intended outcome. Markets and humans are dynamic, responding to change. When Orbitz tasked an algorithm with maximizing revenues, it offered more expensive hotel rooms to Macbook users. When Amazon rolled out Prime in Boston based on purchase history, its data model excluded areas where minorities lived.
Unintended consequences are hard to predict. The US is a litigious society, where many laws are created on precedent and shaped by cases that make their way through the courts. This leads to seemingly ridiculous warnings on packaging (so people don’t eat laundry pods, for example.)
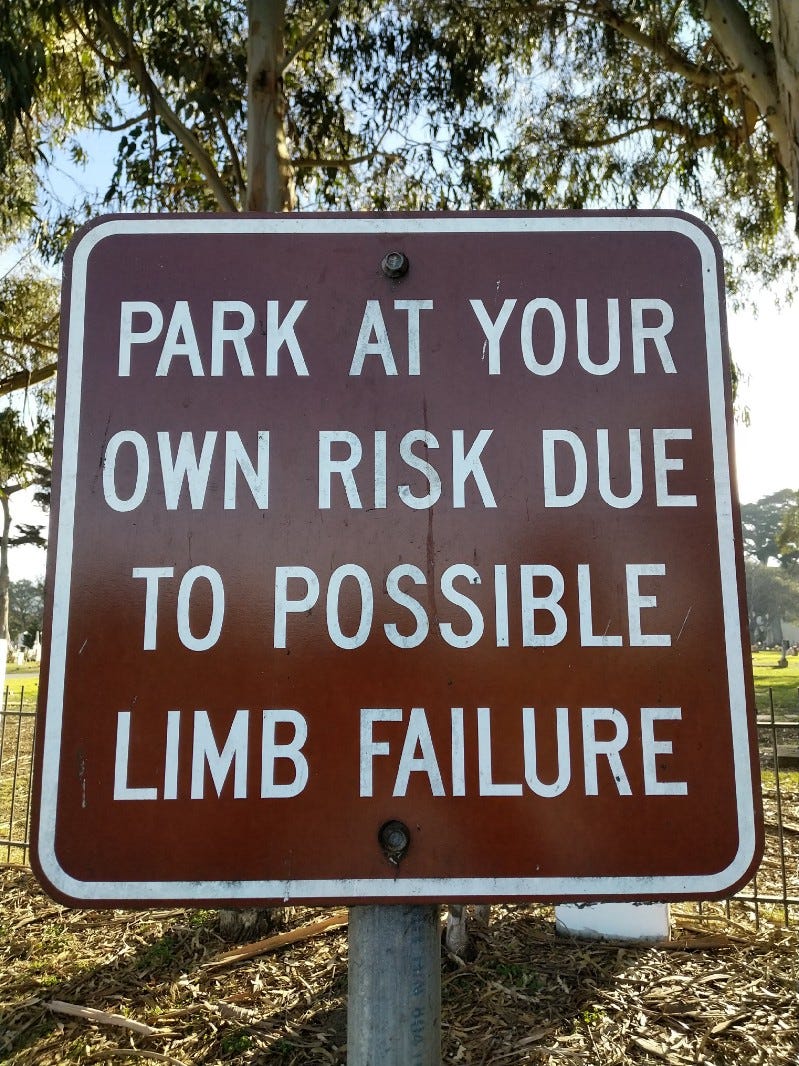
Easy to misinterpret.
Liability matters. Companies I’ve spoken to in North America trust their data — perhaps too much. They worry less about using clouds to process private data, or about whether a particular merge is ethical.
But they worry a lot about the consequences of acting on it.
Three parts, one whole
As I said in the outset, this is a very subjective view of the patterns I’ve seen across countries. The plural of anecdote is not data; caveat emptor. But I’ve fielded literally hundreds of questions from audiences both overseas and online; this led me to ask people in each country whether my feelings could be explained by cultural, technical, political, or economic factors.
The reality is, any solid data science plan needs to worry about veracity, storage, analysis, and use. There are plenty of ways cognitive bias, technical error, or the wrong model can undermine the way data is put to use. Critical thinking at every stage of the process is the best answer, regardless of country or culture.